The three data pillars for building a comprehensive credit operation
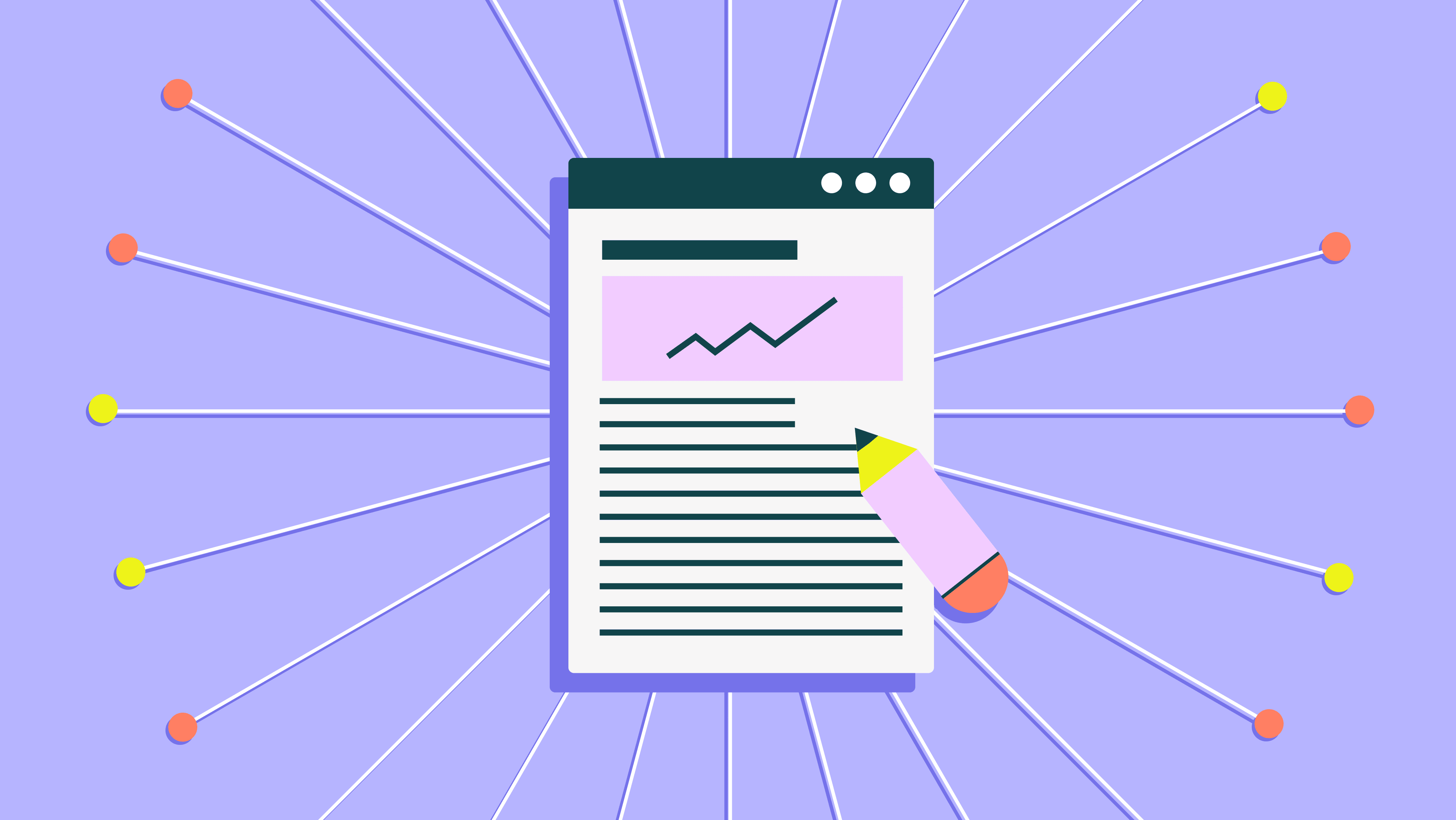
In an age where data is collected and traded as a commodity, the important skill in credit underwriting is not necessarily finding it, but rather knowing how to isolate the most compelling elements.
Data is the foundation of decision making. Without data, our decisions are simply guesswork. And that’s the last thing you want when underwriting credit…
Naturally, the more data you have, the more sound decisions you can reach. But in an age where data is collected in abundance and is traded like a commodity, the important skill is not necessarily finding it, but rather knowing how to review and sift through it to isolate its most compelling elements.
This is particularly true in credit underwriting: anyone extending credit needs to prioritize the most revealing data, or data combinations, in order to determine and quantify the risk in extending credit, within budget.
The trinity of data
In credit underwriting there are hundreds of sources to tap into to find data about potential borrowers. I tend to group these into three main categories:
- Traditional 3rd party data providers
- Next-gen 3rd party data providers
- Proprietary data
Let’s start by talking a little about what each of these actually mean.
Traditional data providers
Traditional data providers are established businesses, providing structured, concise, robust information. These sources include different credit bureaus such as Experian, Dun & Bradstreet or Schufa. They can provide both consumer as well as business data for credit underwriting and other purposes.
Traditional data providers’ data is highly regulated, which makes it reliable and dependable. In many cases, they deliver added value beyond raw information (and an abundance of it) in the form of aggregated scores - calculated by their sophisticated models. These scores provide the bureau’s prediction of the customer’s financial strength and stability, for example: the likelihood of repaying a loan in the ensuing 12 months.
Next-gen data providers
While traditional data sources have been around for decades, the new digital economy has led to new types of providers - ones that don’t just rely on credit, but tap into banking, accounting, payroll, eCommerce platforms and more. These are sources like Plaid, Codat, Enigma, Rutter and the likes. These are the “new” kids on the block (even though some have been around for a while). They offer a different look at financial behavior and status, but most require explicit permission from your applicant for you to review their data. Whilst traditional sources might also require permissions, the friction is generally more significant with next-gen providers - and that has implications on customer experience which need to be considered.
On top of these providers, we can add a newer segment of providers to the mix - providers who contextualize and arrange data, so that you can easily pick out the insights. Think of the output from a service like Plaid. You can receive data on hundreds of bank transactions, and they’re even tagged, but how time consuming and resource intensive is it to glean insights to obtain an accurate financial snapshot? Wouldn’t it be great to get a clean file that shows how the account is actually doing?
New services like Heron and Ocrolus can arrange mounds of data to provide the insights needed on a silver platter.
Proprietary data
Potentially the best type of data, which is most likely the hardest to get early on, is right at your fingertips. Documenting your business interactions with your customers over the years is what some consider the holy grail of data. Unlike traditional and next-gen sources, this is something which only you have, and is tailored to your business. This can include payment history and volumes, line history, repayment schedules, and other metrics that are highly useful in credit underwriting and monitoring. So if you have data, you definitely need to integrate your sources with any decisioning platform.
To ensure optimal usage of proprietary data, it is critical to store and tag it properly, promote analysis and derive the most of what’s yours with little or no cost.
What data will best serve your business?
There is no textbook answer to the above question. Here are some ways to ponder it.
By business stage
The relevance and weight of each source is a direct function of where you are in your business lifecycle. The most obvious example: you’re a brand new business, you’ve taken the strategic decision to extend credit to your customer. You obviously have very little, or no proprietary data of your own - so you need solid, sound data points to assess creditworthiness. Young companies certainly need to rely on established and next-gen providers.
As your business grows and you accumulate more data, it’ll become easier to create reliable models adjusted to your business needs, based on your own proprietary data. You will be able to focus more on your relationship with the customer to determine whether to extend credit - and how much of it, based mainly on past experiences within your system. Of course, you can still rely on established and next-gen sources to alert you on any event occurring to your customer outside your own ecosystem.
By segment (consumer credit vs business credit)
Another determining factor for choosing the best-fitting data sources is the segment to which you’re extending credit.
Consumer credit is heavily regulated. Therefore, readily available data is more robust and accurate. The scores they provide will accurately reflect any past issues that may imply greater risk in extending credit.
Business credit is mostly dependent on a creditor's disclosures, which introduces some consistency issues. While tapping into traditional sources is important and can be very telling, you may be missing some of the story. For commercial credit it makes a lot of sense to tap into newer data sources that can provide information on an applicant’s balance sheets.
By applicant characteristics
Aa a follow up on segment, your applicant characteristics have an important role in deciding the right mix of data sources.
New businesses or younger consumers have fewer records in traditional data sources, as they haven’t accumulated a credit track record. You’ll find empty or thin files, which means you’ll have little to work with. If you’re dealing with new businesses or young consumers, you’ll need to tap into whatever you can find - and that’s where next-gen sources can be a good starting point to making initial decisions. Seeing your customer has a consistent payroll from the same employer over time, or having a high bank account balance for months are some examples which can assist in thin files examples. So seeking these sources will help you serve more customers which are otherwise underserved.
On the business credit side, every industry has its own unique traits, and finding the right data source to reflect the intricacies of the industry you’re working in will help build a robust model that fits your underwriting needs.
By budget
There are no free meals, and the same applies to buying data. Just like marketing teams that are measured by Customer Acquisition Costs, you too are being measured on multiple metrics, data cost being one of them. But data sources are expensive! It’s important to acquire the tools to make the right choices on who to approve and for which product, while ensuring that you’re staying within budget. Your credit operation can’t lose your money. Nor should it only be sustainable. It should be profitable and to let your business grow and scale.
Reseller agreements, such as the ones Noble offers, are a win-win situation. You can get the data you need at a discount rate, and remove long due-diligence processes to help you get your business off the ground fast.
Proprietary data has little or no cost and is pivotal for every business that wants to grow. Sure, early stage businesses can’t really tap into this source off the bat, but building your infrastructure in the right way will also help you lower costs in the mid-long run, something every CEO and CFO loves to hear.
When using your own proprietary data
As already stated, having mounds of your own data is terrific, but it needs to be saved and arranged in a way that can be leveraged for underwriting later on.
For example, you shouldn’t settle for just knowing whether a customer paid or defaulted on their loan. There is data that can help paint a more accurate picture. Did they pay back within the original time frame? Were there any extensions granted? Was the payback date moved once? Twice? Three times? These are all important questions to ask and being able to answer them a quarter or a year or two later will add a lot of value when - especially if you need to adjust your models. They’ll certainly provide a deeper understanding of an individual’s or a business’ financial situation to help with the underwriting process.
But data structures don’t always support such queries. Your data should serve as a full audit trail, with clear indications of any deviation from the original payments scheme. This way your data is contextualized and can portray the nuances needed to reach the most informed decisions.
Balancing opportunity, risk and cost
Tapping into an endless amount of data sources will drive accuracy. But it will also raise costs and eat away at your margins. It’s critical to find the right balance and leverage the most telling data sources to optimize your credit operation.
Traditional sources are dependable, accurate. But they’re also updated slowly, and are less favorable for commercial credit and younger lenders - be they consumers or businesses.
Next-gen sources are sophisticated and revealing. But they’re harder to manage, as the data is not spoon fed - and you’ll often need to rely on your own resources, or other services to decipher the data they provide. They also add friction to the customer journey, as your customers will need to approve access. Lastly, many times these are a single snapshot and not a holistic view of the customer, and can be misleading if used without context and additional sources.
Your own data is a goldmine when it comes to learning about your customers’ financial resilience. But it takes time, precious time to get to a place where it’s matured to be of value, and of course it has its blindspot: there is plenty to learn about an applicant’s financial conduct outside your business and your ecosystem.
The different data sources have their advantages. And the only disadvantage is that using them is costly. So finding the right mix and feeling comfortable (and safe) with it should be a top priority.
To learn more about Noble, visit our website.
If you’d like to see our platform in action, book a demo here.